Robot Hearing, Interpretation & Response
- Sylvia Rose
- Apr 17
- 4 min read
Robots communicate with humans and understand sounds in seemingly meaningful ways. Microphones and sensors designed to detect sound waves are the basis of robot hearing.

Unlike human ears, which transform sound vibrations into signals sent to the brain, robots use microphones and audio processing algorithms. These components work together to capture and interpret sound.
Directional microphones focus on sounds from certain directions. Arrays of microphones improve sound localization accuracy. They're positioned to capture sound from different angles.
In beamforming, the robot can focus on a specific sound source. It "points" its hearing apparatus toward the speaker and filters out background noise.
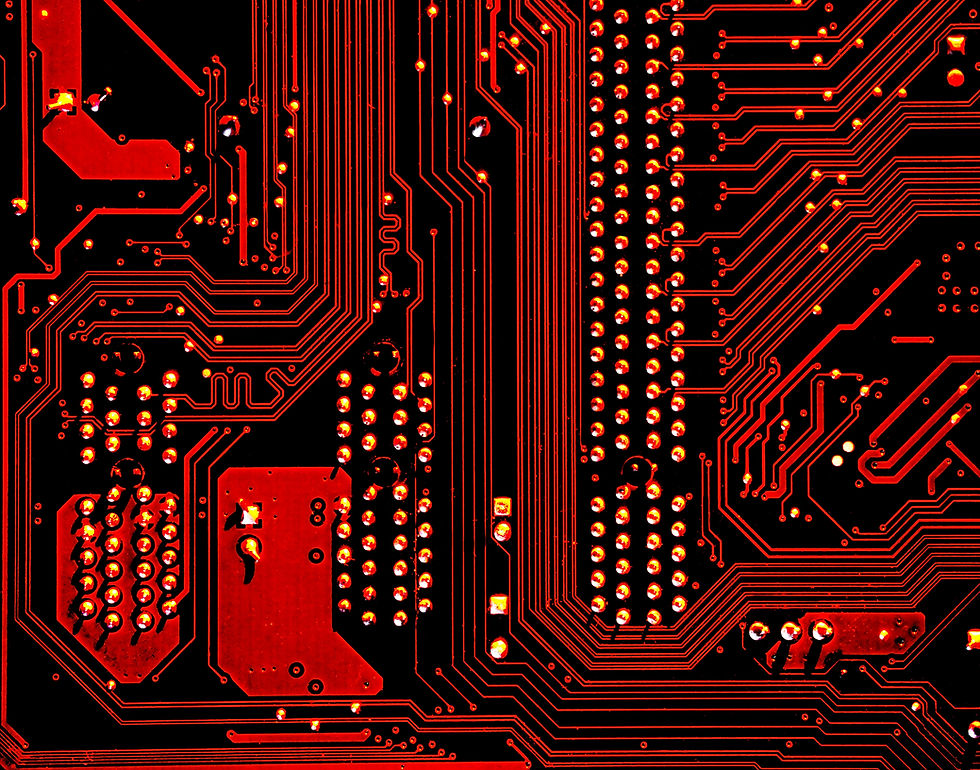
Noise Reduction
Acoustic Echo Cancellation (AEC): For robots which speak and listen simultaneously, AEC prevents the robot's own output from interfering with its ability to hear.
Noise Suppression: Statistical models and machine learning algorithms are used to identify and suppress common background noises, such as fans, humming or clatter.
Speech Recognition & Natural Language Understanding
Once the sound is captured, it's converted into digital signals for analysis. Signal processing algorithms break down the audio into its components: frequency, amplitude and duration.
The digital transformation enables robots to differentiate between sound types, such as human speech, music or environmental noise.

Automatic Speech Recognition (ASR)
ASR is the process of converting spoken language into text.
Feature Extraction: The audio signal is broken down into smaller units called phonemes, the basis of language.
Acoustic Modeling: This uses statistical models, often based on Hidden Markov Models (HMMs) or deep neural networks, to map phonemes to specific words.
Language Modeling: It analyzes the sequence of words to predict the most likely sentence based on grammatical rules and probabilities of word combinations.

Natural Language Understanding (NLU)
This converts the recognized text into a meaningful representation the robot can understand.
Intent Recognition: It identifies the user's goal or intention, such as "turn on the light" or "what is the weather like today?".
Entity Extraction: It identifies specific objects or parameters mentioned in the command, such as "light" or "weather."
Contextual Understanding: It remembers previous interactions to interpret the current command in context.
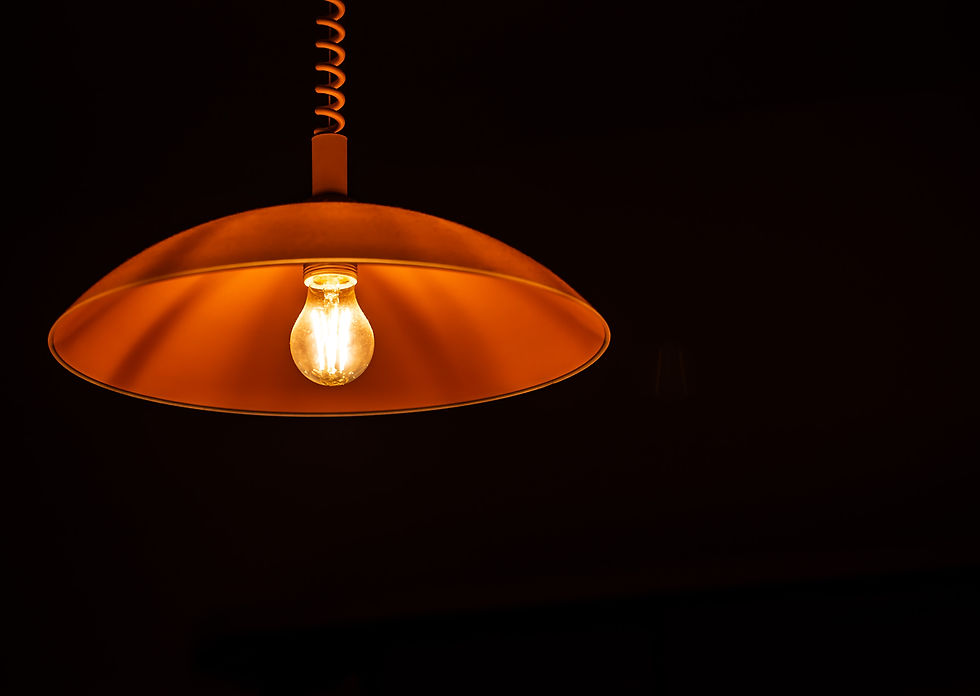
Responding with Words & Actions
Text-to-Speech (TTS): Modern TTS systems use deep learning techniques to generate natural-sounding speech. It's often indistinguishable from a human voice.
Some robots integrate emotional recognition abilities. By analyzing vocal cues like pitch and tone, robots can adapt their responses based on the emotional context.
Factors like intonation, emphasis, and emotion can be incorporated to make the robot's voice more expressive. A TTS system can adjust its voice settings to sound more comforting if it detects heightened emotion.

Action Planning
The bot translates the understood command into a series of actions it can perform.
Movement Control: Manipulating motors and actuators to move the robot's limbs or navigate its environment.
Data Retrieval: Accessing databases or online services to retrieve information, such as the current weather conditions.
Device Control: Interacting with other devices in the environment, such as turning on lights or adjusting the thermostat.

Examples
Voice Assistants: These virtual assistants rely heavily on speech recognition and NLU to respond to voice commands, play music, set alarms and control smart home devices.
Industrial Robots: In manufacturing environments, robots use sound and speech recognition for tasks like voice-controlled programming, error reporting, and collaborative work with human operators.
Social Robots: Robots designed for companionship or therapy use speech recognition and NLU to understand spoken requests, respond emotionally, and engage in conversation.
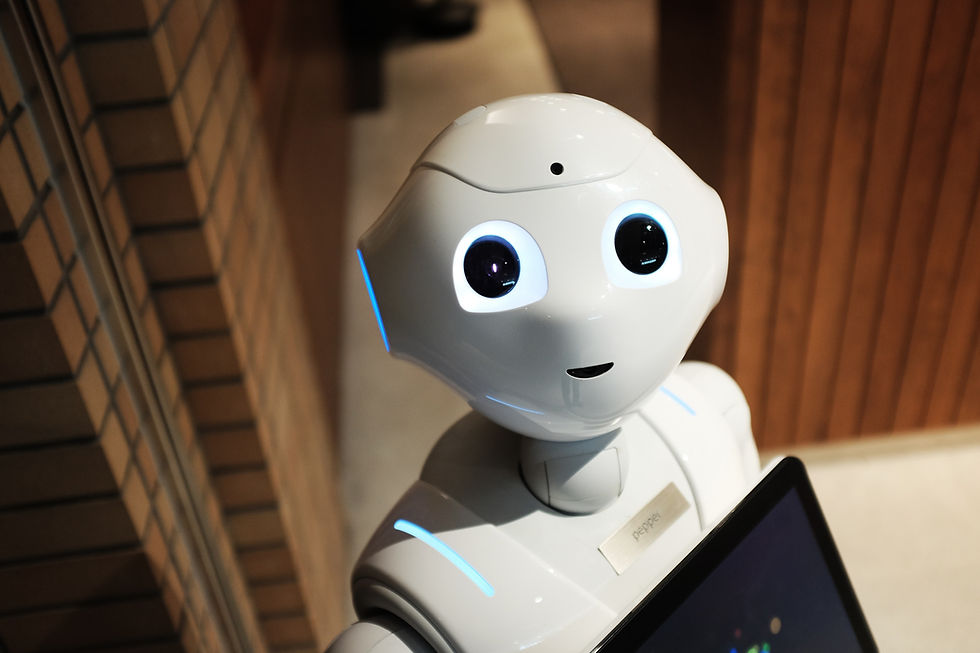
Problems
Robustness to Noise: Improving the accuracy of speech recognition in noisy environments is an ongoing area of research. Robots need to be equipped to filter out unwanted sounds while still recognizing human speech.
Understanding Complex Language: Handling ambiguous language, sarcasm and nuanced requests requires more sophisticated NLU algorithms.
Human language is inherently ambiguous. Many words have multiple meanings depending on context, or different pronunciations.
Cultural Differences: Adapting speech recognition and NLU to different languages and cultural contexts is a current difficulty.
Emotional Intelligence: Developing robots to understand and respond to human emotions is complicated. Understanding emotions is necessary for communication, yet remains a challenge for robots.
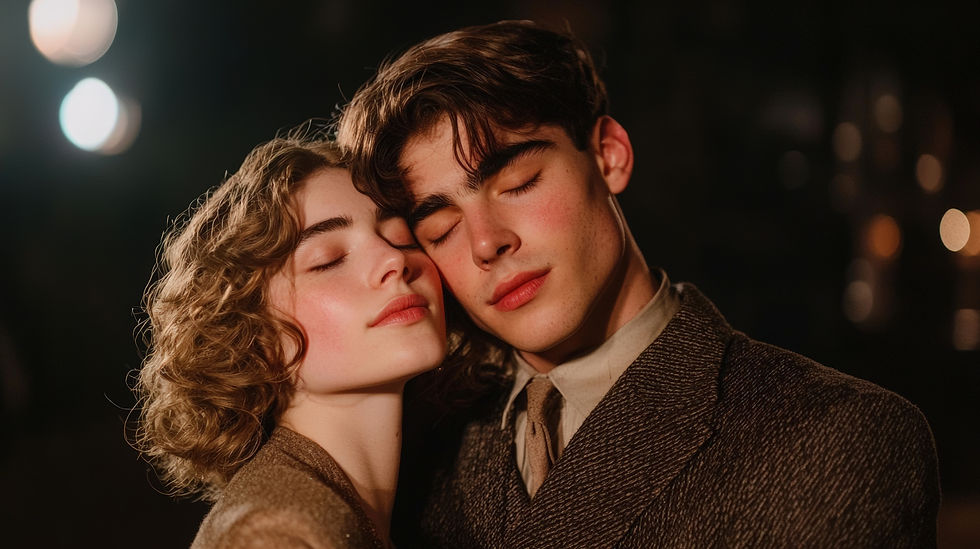
READ: Lora Ley Adventures - Germanic Mythology Fiction Series
READ: Reiker For Hire - Victorian Detective Murder Mysteries